By Marine Jacquemin (Guest Author), 28 January 2025
The European Health Data Space (EHDS) Proposal aims to promote cross-border data sharing within the EU to foster innovation and advance health research. It would be implemented in the form of a federated, cross-border data-sharing infrastructure. While federated learning (FL) is not new, it only recently started being implemented in healthcare research. As such, the ethical and legal implications of its implementation in the EHDS are still uncertain. Studying projects like STRONG-AYA, which uses FL to share data on Adolescent and Young Adults (AYA) with cancer securely and ethically, provides valuable insights.
What is federated learning?
FL is a method that allows the generation of novel insights from decentralized data sources, without needing to centralize data onto a single server. In STRONG-AYA, FL is implemented according to the Personal Health Train (PHT), a framework adapted to health research purposes which uses a train analogy to explain the way it operates. When a partner decides to conduct research, their query (“train”) travels along secure encrypted channels (“train tracks”), and stops at various local databases (“datastations”), where it analyzes local data. These local results will then be aggregated and sent back to the researcher. They thus receive the answer to their query without gaining access to any patient-level data.
Federated learning and GDPR compliance
Because FL allows for patient-level data to remain obscured during analysis, it is often advertised as “privacy-preserving”. Aggregated results generated from federated analyses are usually considered anonymous data that falls outside the scope of GDPR. However, compliance with data protection regulation remains essential in all preceding steps, including data collection, curation and processing on the local level, before the final aggregation. Furthermore, when researching rare diseases, such as AYA cancer, for which data is scarce, FL use might not be enough to guarantee anonymous results, as the primary privacy-enhancing mechanism of FL is aggregation, hiding in numbers. The idea that it could be a magic bullet that would exempt researchers or commercial actors from complying with the GDPR should therefore be dismissed.
Benefits of implementing Federated Learning for health research
While the privacy-preserving claims of FL should be examined with caution, its implementation can still offer significant benefits. Indeed, some of the most pressing ethical and legal concerns associated with big data analytics, namely lack of transparency and loss of control over one’s data, are strongly tied to data centralization. Allowing patients’ information to remain in its original location, without being transferred or copied, could lead to increased visibility and control over one’s own data. Depending on implementation parameters, data owners could view who wishes to access their data and for what purpose, and only then decide whether they will grant or deny access to their data. Going back to our train analogy, this would amount to traffic signals that would allow or forbid the train to stop at one’s data station. Moreover, should a patient decide to withdraw their consent to share their information, tracking that data and ensuring it won’t be accessed again is made much simpler in a federated setting, as it only exists in a single health institution rather than on a database abroad, and/or having been copied elsewhere.
Conclusion
FL opens new opportunities to generate value from our data in a more responsible and ethical manner. However, it remains to be seen how exactly the EHDS will use this technique, and whether it will fully take advantage of the possibilities it offers, or merely use it to counter technical and administrative issues associated with cross-border data sharing.
Keywords: federated learning, European Health Data Space, cancer research, health research, big data, ethics, data protection
Quotes :
While federated learning (FL) is not new, it only recently started being implemented in healthcare research. As such, the ethical and legal implications of its implementation in the EHDS are still uncertain.
Aggregated results generated from federated analyses are usually considered anonymous data that falls outside the scope of GDPR. However, compliance with data protection regulation remains essential in all preceding steps, including data collection, curation and processing on the local level (…)
(…) some of the most pressing ethical and legal concerns associated with big data analytics, namely lack of transparency and loss of control over one’s data, are strongly tied to data centralization.
(…) it remains to be seen how exactly the EHDS will use this technique, and whether it will fully take advantage of the possibilities it offers, or merely use it to counter technical and administrative issues associated with cross-border data sharing.
Picture free right to use
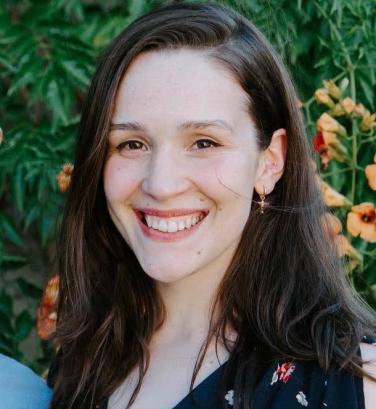
Marine Jacquemin
PhD Research at Clinical Data Science Group
Maastricht University
Email: marine.jacquemin@maastro.nl